Big Data refers to the vast and complex datasets that require specialized tools for efficient management and analysis, which can be overwhelming. But that's where Hadoop comes in. It's like a super tool designed to handle this massive load of data, making it easier to store, process, and analyze. So, when it comes to tackling Big Data challenges, Hadoop is the go-to solution.
Big Data:
Big Data involves handling large-scale structured and unstructured information that organizations generate and manage daily. It's characterized by three main aspects: volume, velocity, and variety. The term 'volume' signifies the enormous quantity of data produced, ranging from small-scale business records to global-scale datasets spanning petabytes and beyond. Velocity relates to the speed at which data is generated and must be processed in real-time to extract valuable insights. Variety encompasses the diverse sources and formats of data, including text, images, videos, social media posts, sensor data, and more.
Big Data analytics is crucial in driving insights and innovation in various industries. For instance, in the retail sector, companies examine past purchases, online browsing patterns, and engagement on social platforms to tailor marketing strategies and enhance customer interactions. In healthcare, Big Data analytics helps identify trends, anticipate potential disease outbreaks, and refine treatment strategies by analyzing extensive patient health records and medical data. Additionally, in finance, banks utilize Big Data analytics to detect fraudulent transactions, assess credit risks, and improve customer service. These real-world examples underscore the transformative impact of Big Data analytics in driving business growth and improving decision-making across industries.
Hadoop:
Hadoop is a powerful framework designed to address the challenges of processing and analyzing large volumes of data. Originating from Apache, Hadoop was created to handle the massive amounts of data generated by web companies like Google, Yahoo, and Facebook. Its primary purpose is to enable efficient storage, processing, and analysis of Big Data.
At the core of Hadoop are two key components: Hadoop Distributed File System (HDFS) and MapReduce. HDFS is a distributed file system that provides reliable and scalable storage for large datasets across clusters of commodity hardware. HDFS divides extensive files into manageable chunks, spreading them across several nodes within a cluster to enhance fault tolerance and ensure continuous availability.
MapReduce is a programming model and processing engine used for parallel processing of data across distributed clusters. It divides data processing tasks into smaller sub-tasks, which are executed in parallel on different nodes in the cluster. MapReduce facilitates efficient computation by distributing data and processing tasks closer to where the data resides, minimizing data movement across the network.
Hadoop's distributed architecture enables the parallel processing of large datasets by distributing data and computation across multiple nodes in a cluster. This parallel processing capability allows Hadoop to efficiently handle massive volumes of data by leveraging the combined computing power of the cluster's nodes. Additionally, Hadoop's distributed nature provides scalability, fault tolerance, and high availability, making it well-suited for processing Big Data on clusters of commodity hardware.
Core Components of Hadoop:
1. Hadoop Distributed File System (HDFS):
Hadoop Distributed File System (HDFS) is the primary storage system for Hadoop, and it is dedicated to storing large files across multiple nodes in a distributed manner. HDFS serves as Hadoop's core storage system, designed to manage large datasets by dividing them into smaller segments, typically between 64 MB and 128 MB. These segments are then spread across multiple nodes within the cluster. To maintain reliability, HDFS duplicates each segment across different nodes, ensuring data recovery in case of hardware failures. Furthermore, its scalable architecture allows organizations to increase storage capacity effortlessly by integrating additional nodes into the system. Moreover, HDFS provides high throughput for data access and processing, as it allows parallel read and write operations across multiple nodes, leveraging the aggregate bandwidth of the cluster.
2. MapReduce:
MapReduce is a computational framework within Hadoop that enables the parallel processing of extensive datasets across distributed systems. The MapReduce programming model divides data processing tasks into two main phases: map and reduce. In the map phase, input data is divided into smaller chunks and processed independently in parallel across multiple nodes in the Hadoop cluster. Each node applies a user-defined map function to the input data, transforming it into key-value pairs. In the reduce phase, the intermediate key-value pairs produced by the map phase are shuffled, sorted, and grouped by key, and then processed by user-defined reduce functions to generate the final output.
An example of a MapReduce job could involve processing a large log file to count the occurrences of each word. In the map phase, each node reads a portion of the log file and emits key-value pairs, where the key is a word and the value is the count of occurrences. In the reduce phase, the intermediate key-value pairs are aggregated by word and summed up to produce the total count of each word across the entire log file. This parallel processing approach allows MapReduce to efficiently handle large-scale data processing tasks by leveraging the distributed computing capabilities of the Hadoop cluster.
The Architecture of Hadoop:
The architecture of Hadoop comprises several key components that work together to enable distributed storage and processing of large datasets. At its core, Hadoop consists of two main components: Hadoop Distributed File System (HDFS) and Yet Another Resource Negotiator (YARN). HDFS is responsible for storing and managing data across multiple nodes in the Hadoop cluster, while YARN is the resource management framework responsible for allocating system resources and scheduling tasks across the cluster.
In a Hadoop cluster architecture, there is a hierarchical structure consisting of master and slave nodes:
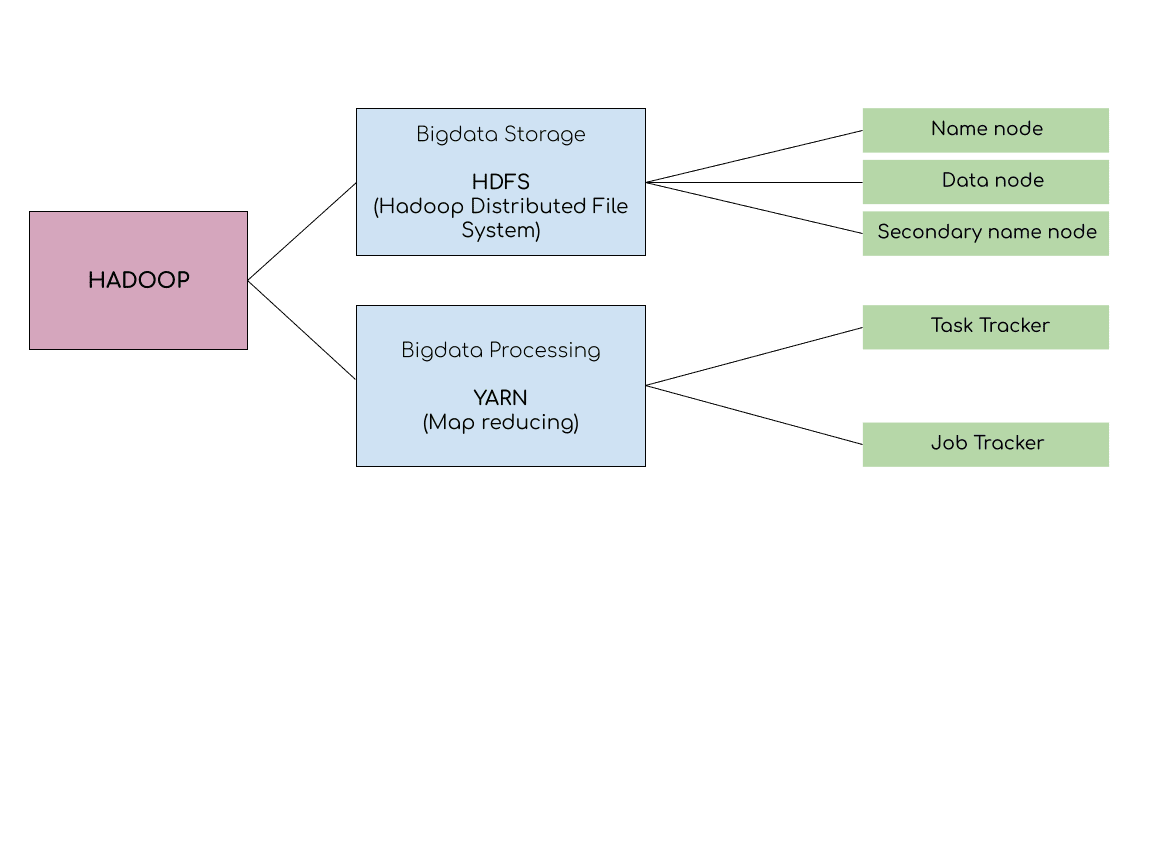
The Interaction Between NameNode and DataNodes
HDFS follows a master-slave architecture, where the NameNode acts as the master and DataNodes function as slaves. The NameNode is the central server responsible for managing the file system's metadata, which includes information about file structure, file locations, and block mappings. However, the NameNode does not store the actual data; instead, it keeps track of where each piece of data is stored across the cluster. The DataNodes, on the other hand, are responsible for storing the actual data blocks. Each DataNode manages its storage and regularly communicates with the NameNode to report the status of its stored blocks. This communication is crucial for maintaining data integrity and ensuring that the data is available even in the event of hardware failures.
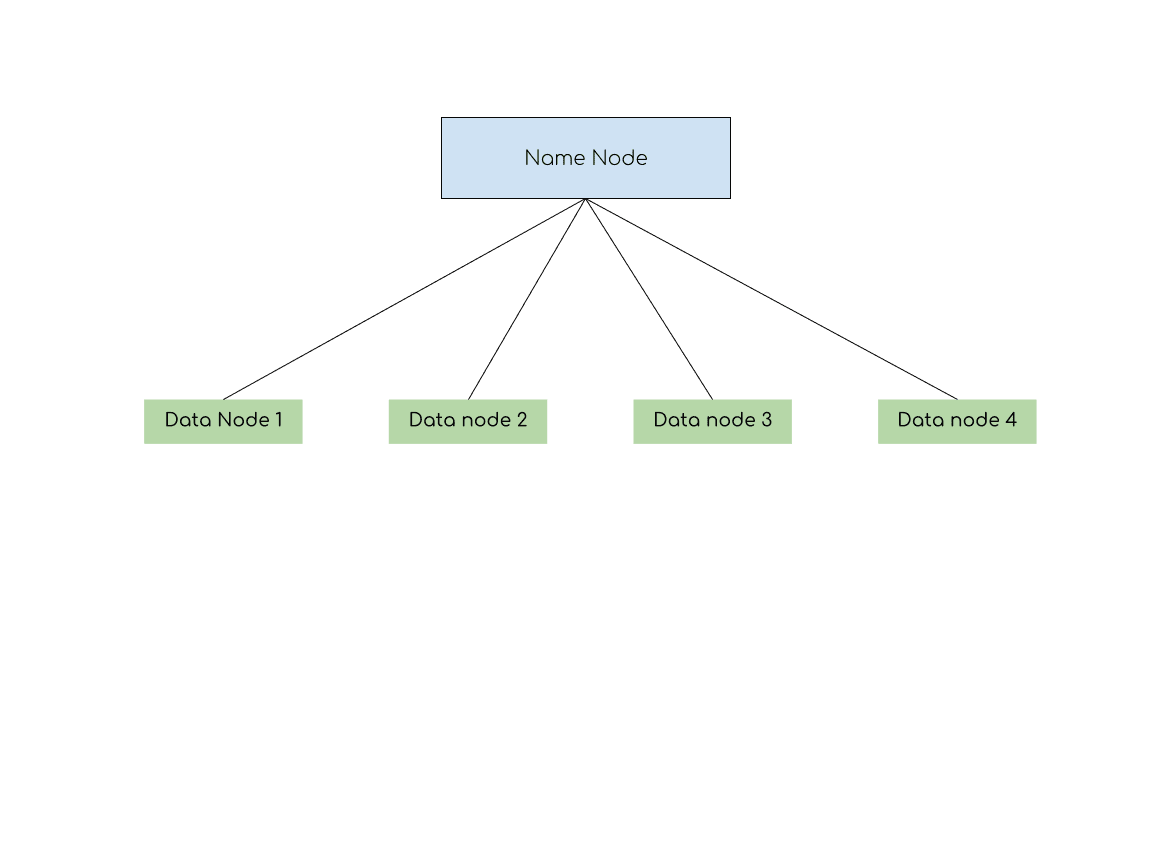
When a file is uploaded to HDFS, the NameNode divides it into smaller blocks, typically 128MB each. These blocks are then distributed across multiple DataNodes. Several factors influence the decision of where to place these blocks:
* Location: The NameNode prioritizes storing data blocks on DataNodes that are geographically or network-wise closest to the client or the source of the data. This reduces latency and increases data access speeds.
* Network Traffic: Before allocating data to a DataNode, the NameNode checks the network traffic to ensure that the chosen node is not currently overloaded. This helps in balancing the load across the cluster and preventing bottlenecks.
* Data Redundancy: To ensure reliability, HDFS replicates each data block across multiple DataNodes. The default replication factor is three, meaning each block is stored on three different DataNodes. The NameNode ensures that these replicas are placed on different racks or locations to safeguard against data loss in case of hardware failure.
The continuous communication between the NameNode and DataNodes is maintained through a mechanism called the heartbeat. Every three seconds, DataNodes send a heartbeat signal to the NameNode to confirm that they are functioning correctly. This signal does not carry any data but is a crucial indicator of the node's health.
If the NameNode does not receive a heartbeat from a DataNode within a specified timeframe, it assumes that the node has failed. In response, the NameNode triggers a data redundancy process, where the missing blocks are replicated to other DataNodes to maintain the required replication factor.
The heartbeat mechanism also helps the NameNode monitor network traffic. If a DataNode's response time increases, indicating potential network congestion or hardware issues, the NameNode may choose to reallocate new data blocks to less congested nodes, ensuring the cluster's overall performance remains optimal.
Architecture of Name Node
The NameNode manages the metadata of the Hadoop Distributed File System (HDFS), while the Secondary NameNode plays a supporting role, periodically backing up the metadata to prevent data loss in case of NameNode failure. To secure communication between the NameNode and the Secondary NameNode, public-key encryption is used.
Public-Key Encryption Mechanism
Public-key encryption ensures secure communication over the network. In this system:
* Each node has a public key and a private key.
* The public key is shared openly and can be used by others to encrypt messages, but the private key remains confidential and is used to decrypt the data.
With this setup, even if a message encrypted with the NameNode's public key is intercepted, it cannot be decrypted without the corresponding private key, which only the NameNode possesses.
Backup Process of the Secondary NameNode
The Secondary NameNode requests permission from the NameNode to back up metadata. The request is encrypted with the public key of the NameNode. Upon receiving the request, the NameNode decrypts it with its private key, verifying the authenticity of the request. If validated, the NameNode encrypts its permission response using the public key of the Secondary NameNode. The Secondary NameNode decrypts this response to confirm it has received permission. To further verify the legitimacy of the Secondary NameNode, the NameNode generates random values (unique identifiers) and sends them to both the Secondary NameNode and the DataNodes. The Secondary NameNode forwards these values to the DataNodes. If the random values match, the Secondary NameNode is allowed to collect the metadata. If they do not match, the DataNodes notify the NameNode, which will then deny access, helping to detect any unauthorized attempt.
Data Redundancy and Replication Factor
The Secondary NameNode stores redundant copies of metadata from the NameNode to safeguard against data loss. The
replication factor defines the number of copies of each data block stored across the nodes:
* For example, if the replication factor is set to 5, five copies of each data block will be stored across different DataNodes.
* In Hadoop, the default replication factor for metadata backup is 1, while in YARN (Yet Another Resource Negotiator), the default replication factor is 3.
Rack Concept for Backup
To improve reliability, the Secondary NameNode distributes copies across multiple racks. Once the initial backup copy is created, additional copies are made from previous backups, ensuring that data is not lost in case of rack failure. This setup helps HDFS maintain data integrity and availability, even in large clusters.
Architecture of Job Tracker and Task Tracker
The JobTracker and TaskTracker follow a master-slave architecture, where a single JobTracker (Master) coordinates multiple TaskTrackers (Slaves). Communication between the JobTracker and TaskTrackers occurs through a heartbeat signal, which is sent every 3 seconds to ensure system health and availability.
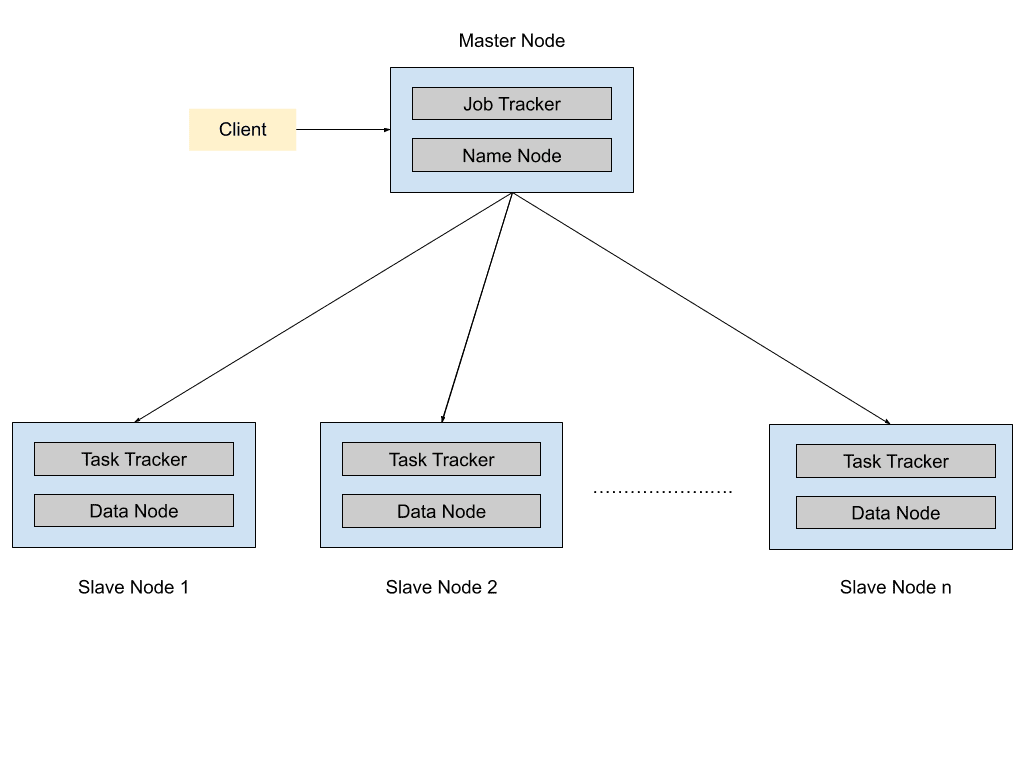
Communication Mechanism
* Master-to-Master Communication: The JobTracker communicates with the NameNode (NN) to retrieve metadata about the data blocks.
* Slave-to-Slave Communication: TaskTrackers interact with DataNodes (DN) to fetch the required data for processing.
* Master-to-Slave Communication is NOT Direct: Instead, the JobTracker assigns tasks to the TaskTrackers after gathering metadata from the NameNode.
Workflow of Job Execution
1. Job Assignment:
* The JobTracker retrieves metadata from the NameNode (Master-to-Master).
* Based on the available resources, the JobTracker assigns tasks to TaskTrackers that have less workload.
2. Data Processing:
* TaskTrackers, upon receiving the task, fetch data from the corresponding DataNodes (Slave-to-Slave).
* Processing occurs only at the TaskTracker, and no storage happens here.
3. Result Transmission & Storage:
* TaskTrackers return the processed results to the JobTracker.
* The JobTracker sends this final output to the NameNode.
* The NameNode stores the result by distributing it across DataNodes following its standard HDFS storage mechanism.
This architecture ensures efficient distributed task execution, where computation is performed as close as possible to the data to reduce network overhead.
To generalize, understanding Hadoop’s architecture and components is essential for managing big data efficiently. It provides a scalable, fault-tolerant framework for distributed data processing.
To read more about How to Install Hadoop on Ubuntu, refer to our blog How to Install Hadoop on Ubuntu.